Thermo Fisher Scientific Inc says the Center for Experimental Bioinformatics (CEBI) at the University of Southern Denmark is using Thermo Scientific nano-ultra high performance liquid chromatography (UHPLC) technology to accelerate productivity and improve efficiencies during its proteomics research.
Implementation of the Thermo Scientific EASY-nLC 1000 has enabled 34 percent more peptide and 22 percent more protein identifications within the CEBI laboratory, significantly increasing productivity. Additionally, the instrument has improved separations and facilitated the analysis of more complex samples within the laboratory.
Based in Odense, the University of Southern Denmark’s Department of Biochemistry and Molecular Biology is world-renowned in the field of proteomics, with research focusing on gene structure and organization, regulation and RNA translation. CEBI is a specialist proteomics research laboratory, aimed at applying modern methods of mass spectrometry and proteomics to functional analysis of genes.
Prior to implementing nano-UHPLC technology, CEBI used conventional HPLC. However they needed a more complete solution, such as EASY-nLC 1000, to accelerate productivity and improve efficiencies. The EASY-nLC 1000 is used in conjunction with Thermo Scientific ion trap and Orbitrap mass spectrometers for a wide range of proteomics research, including the study of complex mouse dendritic cells.
Using the EASY-nLC 1000 has allowed CEBI to take advantage of the ability to facilitate dedicated separation of biomolecules at ultra-high pressures. The higher operating pressures provided by the EASY-nLC 1000 have increased the number of peptide and protein identifications performed within the laboratory by 34 percent and 22 percent respectively. The instrument also allows selection of the most appropriate column dimensions and solid phase materials in order to identify the most suitable configuration to match analytical requirements. Using thinner, longer columns and smaller beads has improved separation and ionization, enhancing sensitivity and allowing the CEBI team to scale down experiments and reduce costs.
“The EASY-nLC 1000 is a highly robust instrument offering nano-UHPLC performance dedicated to proteomics scientists,” said Lasse Falkenby, research assistant at CEBI. “We decided to implement the new system in our laboratory as we felt that it would revolutionise the way we perform LC-MS analyses. The instrument has allowed our laboratory to increase productivity considerably in a way that would never have been possible before. Implementation was a very smooth process requiring minimal configuration effort and the Thermo Fisher Scientific team of experts provided valuable support both throughout and after this process.”
For more information, visit www.thermoscientific.com/easylc
Exploring science is typically characterized by a lot of puzzles, frustrations or even failures. This weblog is mainly intended to record my working, thinking and knowledge acquisitions. I expect that some reflection would refresh my mind from time to time, and motivate me to move further, and hopefully give me a better view about even changing the landscape of bioinformatics. You are welcome to leave some comments, good or bad, but hopefully something constructive. Enjoy your surfing!
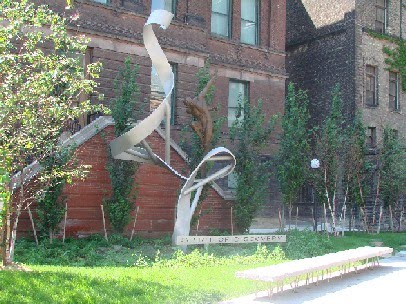
Saturday, November 26, 2011
Thursday, November 17, 2011
Pico Computing Demonstrates Bioinformatics Acceleration at SC 2011
Pico's SC5 FPGA cluster reduces short read sequencing from 6 1/2 hours to just one minute
Seattle, WA (PRWEB) November 09, 2011
Pico Computing will be demonstrating an FPGA implementation of BFAST resulting in a 350X acceleration over a software-only implementation running on two quad core Intel Xeon processors. The 350X acceleration was achieved using 8 Pico M-503 FPGA modules in their SC5 SuperCluster chassis. The BFAST algorithm is primarily used in short read genome mapping.
Read more: http://www.sfgate.com/cgi-bin/article.cgi?f=/g/a/2011/11/09/prweb8945226.DTL#ixzz1dyI5LQs
Labels:
next generation sequencing,
supercomputing
Monday, November 7, 2011
Gene Ontology-driven inference of protein-protein interactions using inducers
Motivation: Protein-protein interactions (PPI) are pivotal for many biological processes and similarity in Gene Ontology (GO) annotation has been found to be one of the strongest indicators for PPI. Most GO-driven algorithms for PPI inference combine machine learning and semantic similarity techniques. We introduce the concept of inducers as a method to integrate both approaches more effectively, leading to superior prediction accuracies.
Results: An inducer (ULCA) in combination with a Random Forest classifier compares favorably to several sequenced-based methods, semantic similarity measures and multi-kernel approaches. On a newly created set of high-quality interaction data, the proposed method achieves high cross-species prediction accuracies (AUC ≤ 0.88), rendering it a valuable companion to sequence-based methods.
Availability: Software and datasets are available athttp://bioinformatics.org.au/go2ppi/
Labels:
algorithms,
gene annotation,
genomics,
PPI,
software
Subscribe to:
Posts (Atom)